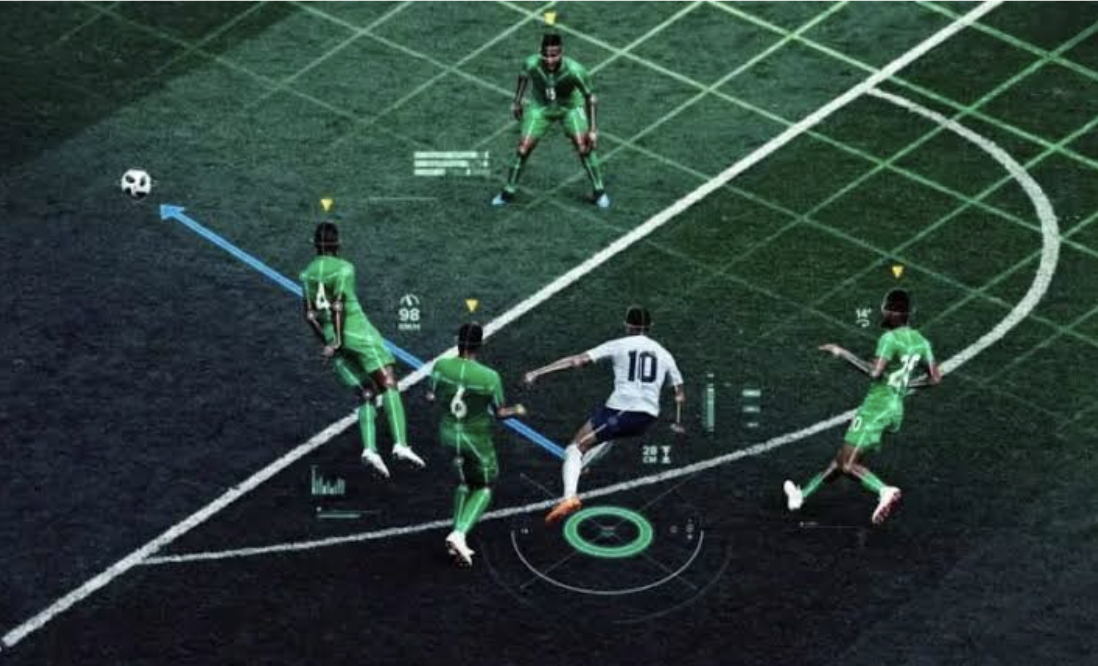
Due to the ability to analyze enormous amounts of data and discover complicated patterns and trends, machine learning algorithms are the newfound methods of achieving accuracy in football predictions today. These algorithms are classified into different types such as supervised learning, unsupervised learning, reinforcement learning, and deep learning. Each type has distinct features and applications in football prediction, and they can be applied to predict player performance, tactical analysis, and injury prediction.
The consequences of using machine learning algorithms in football prediction goes beyond just analysis and coaching decisions, it affects the whole football betting market. For example, football fans and bettors can now use machine learning football tips on this site to make better decisions and improve their betting strategy. However, we recommend understanding the uncertainties and downsides involved with betting and to always proceed with caution while at it.
Types of Machine Learning Algorithms
There are many algorithms that are being adopted for machine learning online. The classification usually depends on how the machine sources data and does its analysis. Some of the most common types include:
- Supervised Learning: These algorithms learn from labeled data, since input features correlate to output labels. In football prediction, supervised learning algorithms use historical data such as player statistics, team performance indicators, and match results to detect patterns and relationships. These patterns can subsequently be used by the algorithms to forecast future match results, player performances, and team strategies. For example, it may evaluate previous matches to estimate a team’s chances of winning based on factors like possession, shots on goal, and past success versus similar opponents.
- Unsupervised Learning: Here, they use unlabeled data to identify underlying patterns or structures. It’s an easy way to group comparable teams or players together based on traits or playing styles. For example, an unsupervised learning system may identify groups of teams that use similar defensive or attacking techniques, offering information about future matchups and outcomes.
- Reinforcement Learning: The technique involves an agent learning to make decisions by interacting with its environment and receiving feedback, such as rewards or penalties. In football prediction, it is often used to create methods (especially for coaches) for improving team performance or player decisions. For example, a reinforcement learning agent may learn to adjust its tactics based on input from past matches, which helps it to improve its predictions for football.
- Deep Learning: These algorithms are modeled after the neural networks seen in the human brain. A typical process here will involve assessing player motions on the field, identifying significant match events, and even providing play-by-play commentary using real-time data. They are ideal for processing complicated, high-dimensional data including photos, audio, and text. They can be used in football prediction to extract valuable insights from massive volumes of data, such as match video footage or text commentary.
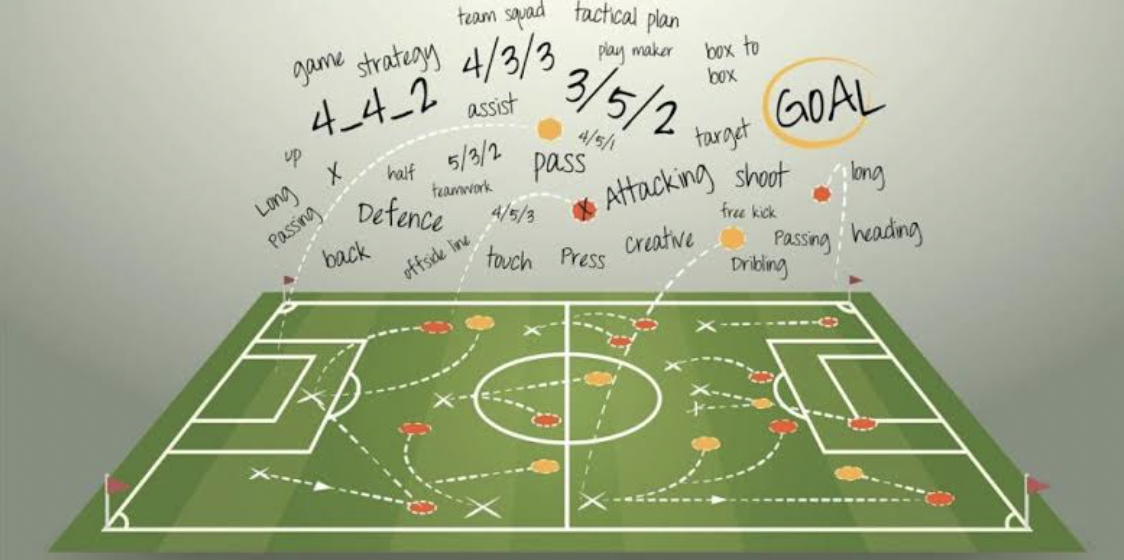
How Machine Learning is Applied in Football Prediction
Different sites and platforms use machine learning and AI for different purposes. But it all borders on the improvement of our prediction accuracies be it for leisure, betting or to improve the overall performance of the teams and players. We’ve explored some of the various ways this technology is being applied and you’ll find some of them quite interesting.
Player Performance
Machine learning algorithms can forecast player performance using historical data, attributes, and contextual factors such as weather, opponent strength, and match importance. Machine learning approaches can identify patterns and trends in player statistics by training models on large datasets. These models can then be used to correctly predict future player performance, allowing coaches to make more informed judgments about player selection, substitutions, and tactical adjustments.
An instance is how they use a player’s previous performance indicators like goals scored, assists, passes completed, and defensive actions to estimate their chances of success in an upcoming encounter. By taking into account characteristics such as recent form, playing position, and previous performance against similar opponents, these algorithms can provide significant insights into which players are likely to have the largest impact on the game.
Team Performance Predictions
Similarly, machine learning algorithms can make accurate football predictions and match team outcomes using team statistics, dynamics, and prior performance. These algorithms can effectively assess opponent teams’ strengths and weaknesses and anticipate match outcomes by examining variables such as squad composition, playing style, possession percentages, shots on goal, and defensive records. This information can help coaches and analysts create game plans, modify tactics, and make strategic decisions to improve their team’s chances of winning.
They can now examine previous encounters between two teams, using criteria such as home advantage, recent form, and head-to-head records to predict whether each side will win, lose, or draw. By taking into account a wide range of variables and incorporating historical data, these algorithms can provide significant insights into match dynamics.
Injury Predictions
Machine learning algorithms have demonstrated potential for predicting player injuries and their impact on team performance. These algorithms can anticipate future player injuries by analyzing data on player fitness, injury history, workload, and recovery time. This information can help coaches manage player workloads, implement injury prevention strategies, and decide on player availability and substitution.
With the right algorithm, information like a player’s training load, physical condition, and injury history can be used to predict their likelihood of injury in an upcoming match. The algorithm would consider characteristics such as fatigue, muscular imbalances, and historical injury patterns; so these algorithms can provide early indications of potential injury risks and assist coaches in taking proactive efforts to reduce them.
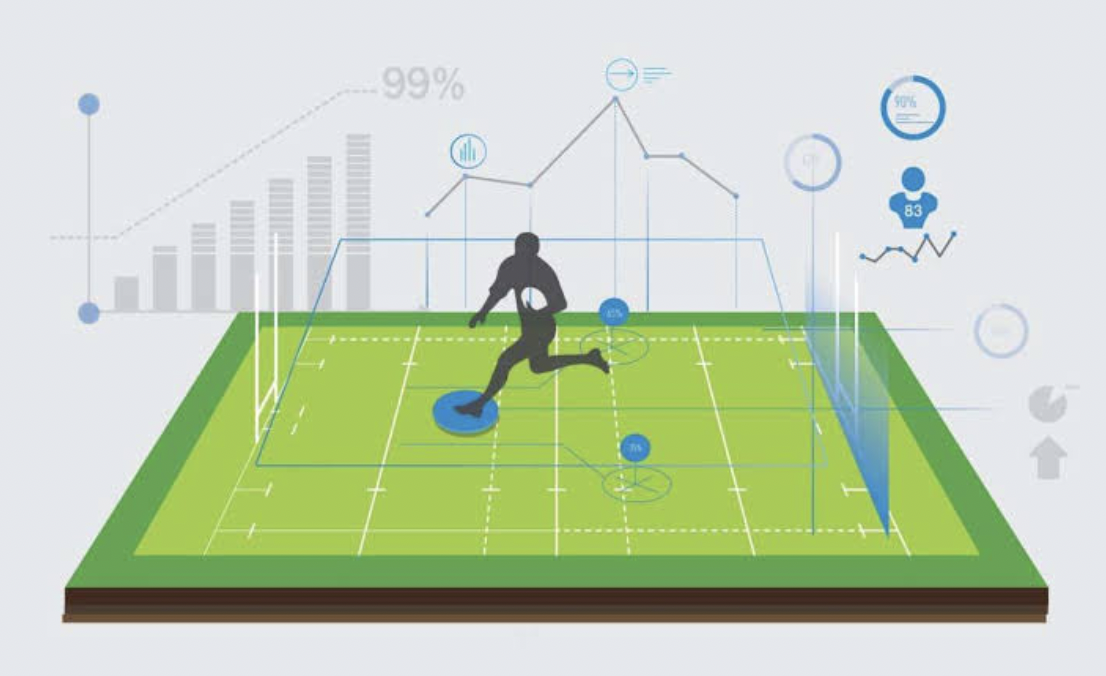
Tactical Analysis
Another application of machine learning in football prediction is tactical analysis, which involves using algorithms to assess tactical methods and game plans in order to improve team performance. By studying match footage, player movements, passing patterns, and defensive formations, machine learning algorithms can discover patterns, trends, and weaknesses in opponents’ tactics and devise plans to exploit them. This information can assist coaches in developing game plans, adjusting formations, and making tactical substitutions to obtain a competitive advantage on the field.
Video footage from previous matches could be used to identify repeated patterns in the opponents’ tactics. So you’ll be able to notice high pressing up the field or quick counter-attacks. These applications of machine learning algorithms allow coaches to devise counter-strategies that neutralize opponents’ strengths while exposing their shortcomings, increasing their team’s chances of victory.
Future of Machine Learning Algorithms In Football Predictions
As technology continues to advance and the methods available for data collection improve, machine learning algorithms will surely follow this trend to become more precise in their predictions. One potential development is to include real-time data streams into machine learning models, so they can make faster and adaptive forecasts during live matches. This will also allow coaches to make real-time tactical adjustments based on predictive data and improve their team’s performance on the field.
Advancements in player monitoring technologies, like wearable devices and camera-based systems, are expected to produce more data streams for machine learning algorithms to analyze. This could result in more precise predictions of player movements, fatigue levels, and injury risks, allowing coaches to improve player performance while lowering the chance of damage.
When we closely observe the soccer betting markets, it’s easy to notice the way accuracy of machine learning forecasts keeps increasing. At this rate, it’s projected to have reached a mind-blowing level where we can perfectly predict future games. So, you should definitely expect better and more accurate football tips and predictions and insights into match outcomes, player performances, and betting odds soon. Which should allow you to make better decisions and potentially increase your chances of winning bets